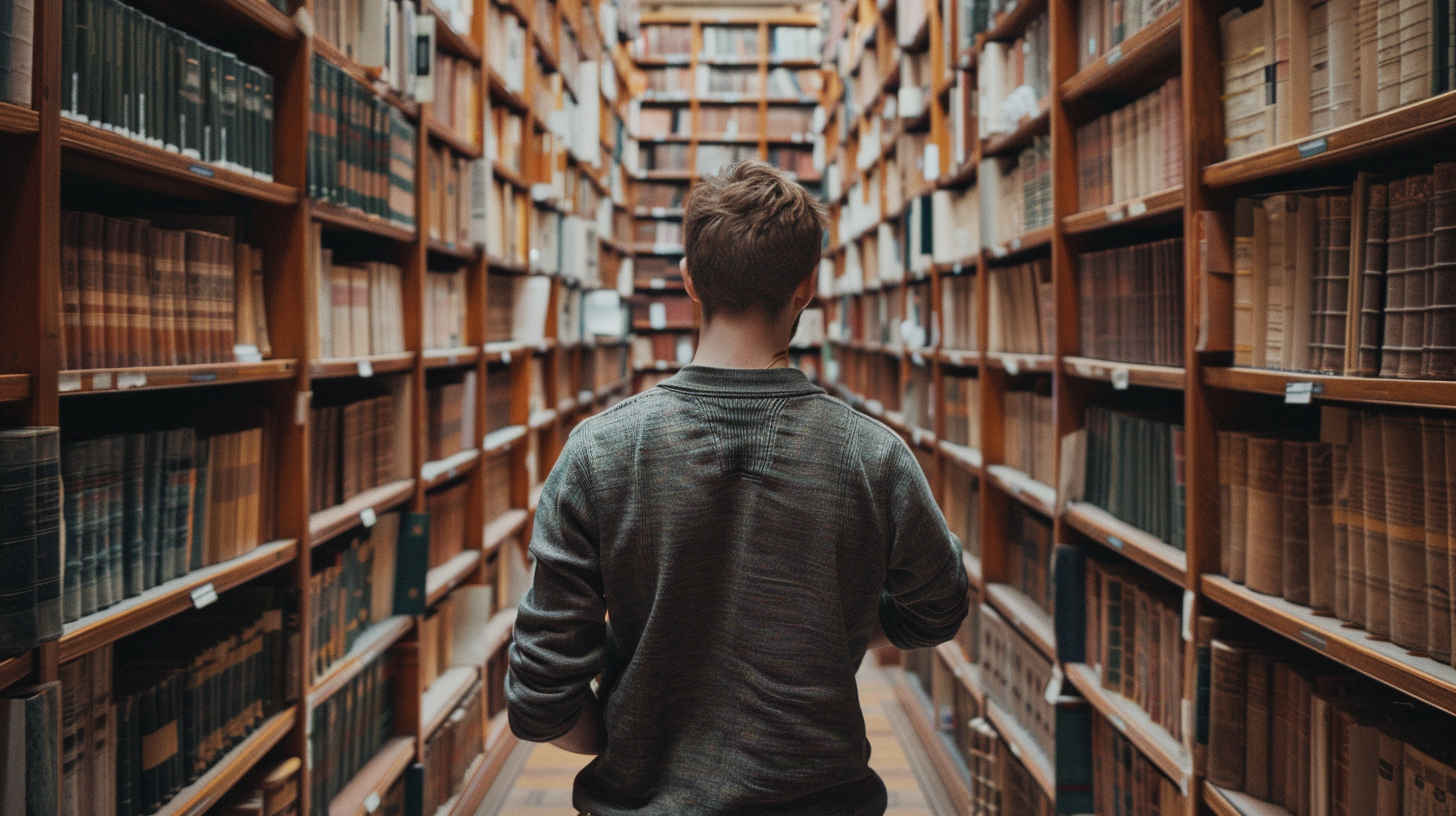
Top AI Research Papers for Enthusiasts
Ilya Sutskever from OpenAI once handed John Carmack a reading list of about 30 research papers, saying, “Master these, and you’ll grasp 90% of what’s crucial in AI today.” To round it out, I’ve added a few more LLM papers to cover the remaining 9%.
Whether you’re passionate about natural language processing (NLP), computer vision, or machine learning, these papers offer invaluable insights into cutting-edge advancements in AI technology.
Must-Read Papers
- Transformers: Attention is All You Need – Explore the groundbreaking transformer model revolutionising NLP.
- BERT: Pre-training of Deep Bidirectional Transformers – Learn about BERT’s impact on language understanding tasks.
- GPT: Language Models are Few-Shot Learners – Understand how GPT models have transformed AI capabilities.
Stay Updated
Stay ahead with the latest AI research and developments. Bookmark this page for ongoing updates and new paper recommendations.
- The Annotated Transformer (nlp.seas.harvard.edu)
- The First Law of Complexodynamics (scottaaronson.blog)
- The Unreasonable Effectiveness of RNNs (karpathy.github.io)
- Understanding LSTM Networks (colah.github.io)
- Recurrent Neural Network Regularization (arxiv.org)
- Keeping Neural Networks Simple by Minimizing the Description Length of the Weights (cs.toronto.edu)
- Pointer Networks (arxiv.org)
- ImageNet Classification with Deep CNNs (proceedings.neurips.cc)
- Order Matters: Sequence to sequence for sets (arxiv.org)
- GPipe: Efficient Training of Giant Neural Networks using Pipeline Parallelism (arxiv.org)
- Deep Residual Learning for Image Recognition (arxiv.org)
- Multi-Scale Context Aggregation by Dilated Convolutions (arxiv.org)
- Neural Quantum Chemistry (arxiv.org)
- Attention Is All You Need (arxiv.org)
- Neural Machine Translation by Jointly Learning to Align and Translate (arxiv.org)
- Identity Mappings in Deep Residual Networks (arxiv.org)
- A Simple NN Module for Relational Reasoning (arxiv.org)
- Variational Lossy Autoencoder (arxiv.org)
- Relational RNNs (arxiv.org)
- Quantifying the Rise and Fall of Complexity in Closed Systems: The Coffee Automaton (arxiv.org)
- Neural Turing Machines (arxiv.org)
- Deep Speech 2: End-to-End Speech Recognition in English and Mandarin (arxiv.org)
- Scaling Laws for Neural LMs (arxiv.org)
- A Tutorial Introduction to the Minimum Description Length Principle (arxiv.org)
- Machine Super Intelligence Dissertation (vetta.org)
- PAGE 434 onwards: Komogrov Complexity (lirmm.fr)
- CS231n Convolutional Neural Networks for Visual Recognition (cs231n.github.io)
- Improving Language Understanding by Generative Pre-Training
- Language Models are Unsupervised Multitask Learners
- Language Models are Few-Shot Learners
- GPT-4 Technical Report
- Llama 2: Open Foundation and Fine-Tuned Chat Models
- What Are Tools Anyway? A Survey from the Language Model Perspective
- Gemini 1.5: Unlocking multimodalunderstanding across millions of tokens of context