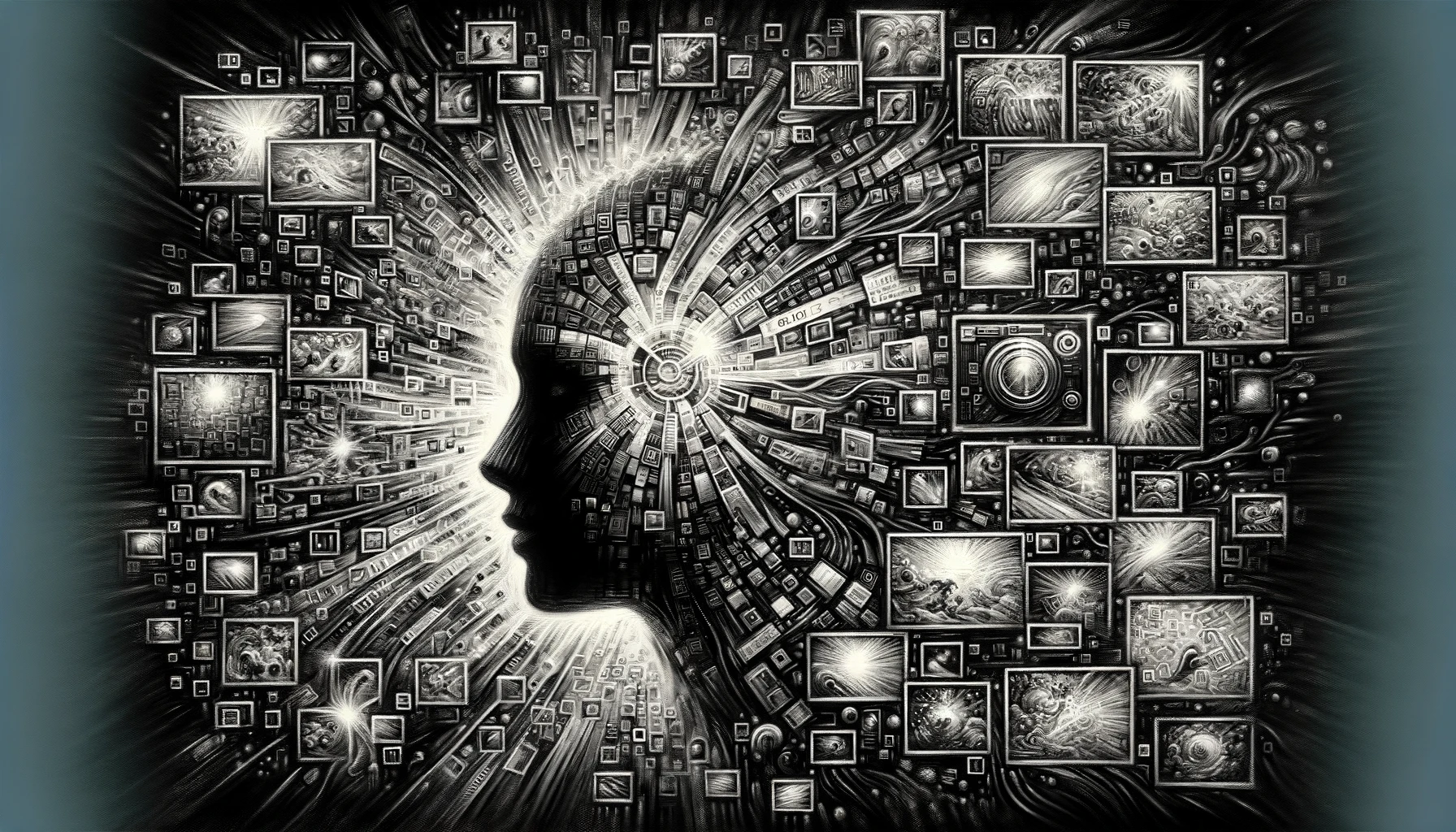
From Text to Reality: OpenAI’s Sora and the Evolution of Video Generation Simulators
In a landmark advancement, OpenAI introduces Sora, its most sophisticated video generation model to date, marking a new milestone in the field of generative models. The release of Sora represents a leap toward building general-purpose simulators of the physical world, harnessing the power of large-scale training across a diverse array of videos and images.
Sora embodies a transformative approach to video modeling, trained on text-conditional diffusion models that operate within a range of durations, resolutions, and aspect ratios. This flexibility allows Sora to generate up to a full minute of high-fidelity video, a feat that demonstrates the significant potential of scaling video generation models.
The technical report detailing Sora’s development emphasizes the methodological innovation behind the model’s ability to turn visual data into a unified representation. This enables large-scale training, echoing the success of large language models which benefitted from internet-scale data training. Sora utilizes visual patches as a scalable and effective representation for training on various types of videos and images, much like tokens are used in language models for diverse modalities of text.
Sora’s architecture is particularly notable for its use of a transformer that operates on spacetime patches of video and image latent codes. This approach allows for the compression of videos into a lower-dimensional latent space, from which spacetime patches are extracted and used as tokens by the transformer. This method of representation facilitates Sora’s ability to train on visual data with variable attributes, making it a generalist model that can cater to a wide range of video and image generation tasks.
The model’s capabilities are not limited to video generation alone. It can also be prompted with pre-existing images or video, enabling a broad spectrum of image and video editing tasks. From animating static images to extending videos in time, Sora’s versatility is a testament to the progress made in the field of AI and machine learning.
One of the more intriguing aspects of Sora’s capabilities lies in its emerging simulation abilities. When scaled, the model exhibits emergent capabilities that simulate some aspects of the physical world without explicit inductive biases. This includes 3D consistency, long-range coherence, object permanence, and even simple interactions that affect the state of the world, like a painter leaving strokes on a canvas.
Despite these advances, Sora does have its limitations. The model does not always accurately simulate the physics of certain interactions, and there are occasional incoherencies in long-duration samples. Nonetheless, these are viewed as challenges to overcome rather than insurmountable obstacles.
OpenAI’s Sora is a step towards an ambitious future where AI can simulate complex aspects of our world. It exemplifies the potential of video models as tools for understanding and interacting with digital and physical environments, showcasing what’s possible when scaling and innovative model architecture come together. As such, Sora is not just a milestone in video generation but a beacon for the future of simulation technologies.